슬롯커뮤니티
슬롯커뮤니티 는 슬롯게임을 중심으로 한 유저 커뮤니티로, 정보 공유, 후기 작성, 이벤트 참여, 팁 공유, 슬롯사이트 추천 등 다양한 활동이 이루어지는 플랫폼입니다. 단순한 슬롯사이트와는 달리 커뮤니티 구성원 간의 양방향 소통이 가능하며, 특히 실시간 후기나 경험담을 바탕으로 신뢰도 높은 정보를 얻을 수 있다는 점이 가장 큰 특징입니다.
또한 슬롯버프, 슬롯타운, 슬롯나라 등 최근에는 커뮤니티 전문 플랫폼들이 늘어나며, 슬롯 유저 중심의 정보 생태계가 더욱 풍부해지고 있습니다.
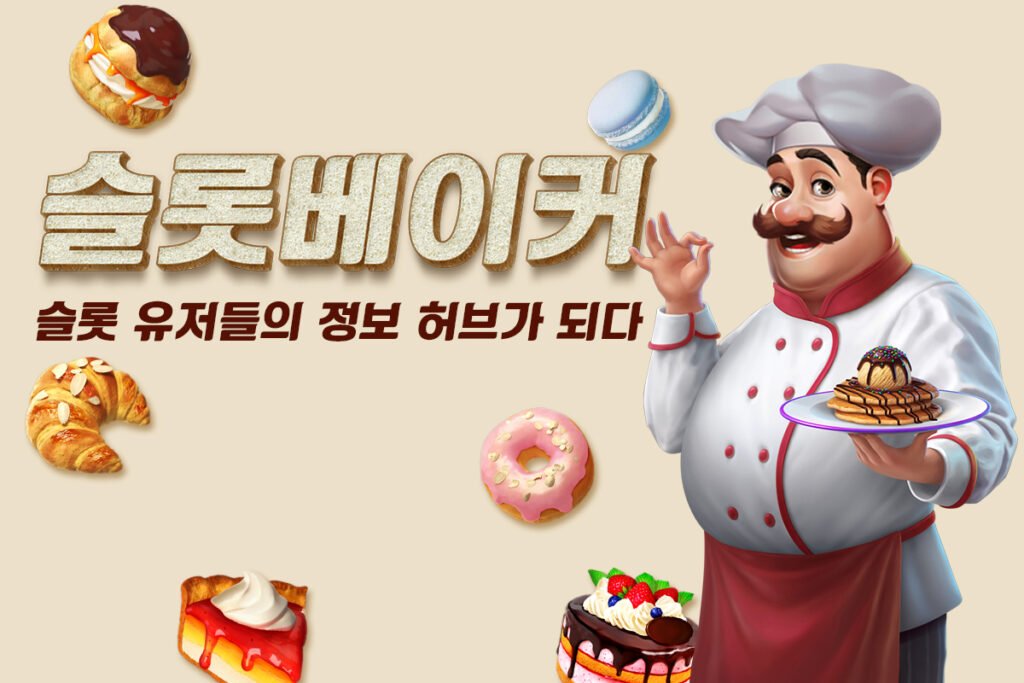
슬롯커뮤니티의 주요 장점
검증된 정보 제공
슬롯커뮤니티는 유저들이 직접 사용해본 슬롯사이트에 대한 후기를 공유하기 때문에, 먹튀 우려가 있는 사이트를 피할 수 있는 정보를 제공합니다. 이를 통해 신규 유저들도 안전하게 슬롯을 즐길 수 있습니다.
실시간 소통
댓글과 게시판 중심의 커뮤니티는 실시간 슬롯 공략, 이벤트 정보 공유, 꽁머니 지급 소식 등 빠른 피드백이 가능해, 단순히 혼자 플레이하는 것보다 훨씬 더 전략적인 플레이가 가능합니다.
이벤트 중심 운영
커뮤니티를 중심으로 한 무료슬롯 대회, 슬롯 리뷰 이벤트, 포인트 리워드 시스템 등은 유저들의 참여도를 높이며, 단순한 게임 이상의 재미를 제공합니다.
대표 슬롯커뮤니티 소개
슬롯커뮤니티 슬롯버프
슬롯 전문 커뮤니티 플랫폼
프라그마틱, PG소프트 공식 이벤트 진행
유저 랭킹 시스템, 리뷰 포인트 지급
무료슬롯챔피언 대회 상시 운영
슬롯나라 슬롯커뮤니티
SEO 정보 중심 아카이브형 커뮤니티
슬롯사이트 순위 정보
슬롯 잘하는 법, 노하우 콘텐츠 특화
슬롯타운 슬롯커뮤니티
데모 체험 & 리뷰 중심 플랫폼
게임사별 인기 슬롯 정리
초보자용 슬롯 체험 가이드 제공
항목 | 슬롯버프 | 슬롯타운 | 슬롯나라 |
---|---|---|---|
주요 특징 | 프라그마틱·PG소프트 공식 제휴, 커뮤니티 포인트 시스템 운영 | 데모 체험 기반의 슬롯 리뷰 콘텐츠 제공 | 슬롯 정보 SEO 콘텐츠 특화, 슬롯사이트 비교 자료 제공 |
이벤트 유무 | 무료슬롯 대회 등 상시 운영 | 체험형 소규모 이벤트 일부 진행 | 정보 제공 중심, 이벤트 없음 |
콘텐츠 성격 | 후기, 공략, 랭킹 중심 | 체험 후기 및 추천 콘텐츠 중심 | 키워드 기반 정보성 콘텐츠 |
타겟 유저층 | 중급~고급 슬롯 유저 | 슬롯 입문자 및 체험 위주 사용자 | 검색 기반 정보 탐색 유저 |
기타 장점 | 랭킹 시스템, 슬롯노하우 공유, 참여형 콘텐츠 풍부 | 게임사별 인기 게임 정리, 초보자용 가이드 제공 | 슬롯사이트 리스트화, 정제된 슬롯 정보 제공 |
이 외에도 슬롯즈, 슬롯피디아 등 다양한 커뮤니티들이 존재하며, 각기 다른 포지셔닝으로 슬롯 유저들의 니즈를 만족시키고 있습니다.
슬롯커뮤니티에서 주로 다뤄지는 콘텐츠
슬롯커뮤니티에서는 다음과 같은 정보들이 자주 업로드됩니다:
실제 슬롯사이트 이용 후기
게임사별 인기 게임 추천
프라그마틱, PG소프트, 플레이앤고 슬롯 소개
슬롯 노하우 및 잘하는 법
무료슬롯 이벤트 참가 팁
슬롯 리스크 관리법
신규 슬롯사이트 소식
특히 초보자들은 이 커뮤니티를 통해 슬롯의 기본 구조와 패턴을 이해하고, 자신에게 맞는 슬롯사이트를 선택할 수 있는 눈을 기를 수 있습니다.
주의해야 할 슬롯커뮤니티의 특징
모든 커뮤니티가 신뢰할 수 있는 것은 아닙니다. 아래와 같은 커뮤니티는 주의가 필요합니다:
과도한 광고 중심 (후기보다 홍보성 콘텐츠 위주)
운영자/파트너 정보 미공개
이벤트가 일회성으로 끝남
안전한 슬롯커뮤니티를 고르기 위해서는 다음과 같은 기준을 참고하면 좋습니다:
유저 활동이 활발하고 실시간 댓글이 존재하는가
게임사 공식 제휴 여부가 명확히 표시되는가
커뮤니티 활동에 따른 보상이 명확한가
운영기간이 6개월 이상 유지되고 있는가
나만의 슬롯 노하우를 공유해보자
슬롯커뮤니티는 단순히 정보를 얻는 공간을 넘어서, 자신만의 슬롯 노하우를 공유하며 존재감을 드러낼 수 있는 무대입니다. 예를 들어 슬롯버프에서는 주간 슬롯 랭킹 시스템을 통해, 유저들의 활동성과 공략법을 포인트화하고 있습니다.
🎯 “당신만의 슬롯 공략법은 무엇인가요?”
댓글 또는 게시판에 남겨주시면 커뮤니티 활동 포인트가 지급됩니다!
이러한 참여형 콘텐츠는 커뮤니티 자체의 생동감을 불어넣고, 유저들 간의 관계 형성을 도와 슬롯 유저들의 장기적인 활동 기반을 만들어냅니다.
마무리: 슬롯 유저라면 커뮤니티를 모르면 손해
슬롯은 단순한 확률 게임이 아니라, 정보력과 경험이 승부를 가르는 게임입니다. 혼자서 무작정 플레이하는 것보다, 수많은 유저들의 실제 경험과 전략이 모인 슬롯커뮤니티를 활용하는 것이 훨씬 현명한 선택입니다.
슬롯사이트 선정, 이벤트 참여, 슬롯 잘하는 법, 프라그마틱 플레이 게임 공략 등 슬롯과 관련된 모든 정보는 커뮤니티 안에 다 들어 있습니다.
슬롯커뮤니티를 선택하는 순간, 슬롯게임은 전혀 다른 레벨로 진입합니다.